Polarimetric Radar simulations with realistic Ice and Snow properties and mulTI-frequeNcy consistency Evaluation (PRISTINE)
Joint project between
University of Cologne and Deutscher Wetterdienst (DWD), Phase 2
University of Cologne: Soumi Dutta (PostDoc), Davide Ori (PI)
DWD: Jana Mendrok (PostDoc), Ulrich Blahak (PI)
Abstract
Project PRISTINE aims to develop a polarimetric radar forward operator that is based on realistic snow particle properties. A reliable forward operator is an essential tool for the overall PROM program since it is a core component of data assimilation algorithms and model evaluation studies. The existing forward operators based on the T-matrix approximation for homogeneous, spheroid-shaped clouds and precipitation particles have shown substantial deviations with respect to the observed polarimetric signatures of frozen hydrometeors (Trömel et al., 2023; see also Fig. 1). Those deviations have been linked to the simplifications introduced by the T-matrix approximation.
PRISTINE is focused on the efficient polarimetric radar simulator EMVORADO, which covers many aspects of radar simulation and is coupled to the weather models COSMO and ICON, but so far applies single-scattering properties based on the T-Matrix approach assuming spheroidal particles for all hydrometeors. The project performs novel single-scattering computations using the Discrete Dipole Approximation (DDA) method. The accurate DDA calculations require additional assumptions regarding the detailed hydrometeor structure. The snow particle shapes will be generated using a combination of detailed Lagrangian cloud modeling and an explicit snow particle simulator. By doing so, PRISTINE aims to find the snow shapes that are representative of the ensemble mean properties of snow. The single-scattering properties are used to replace the cloud ice and snow scattering modules in EMVORADO, which are currently based on the T-Matrix approximation. The performance of the updated scattering simulations will be evaluated against multi-frequency, Doppler-resolved, and polarimetric radar observations.
Figure 1: Comparison of reflectivities (left) and differential reflectivity (right) of homogeneous, spheroidal (y-axis) and realistically shaped (x-axis) models of snow particles. Homogeneous spheroidals match copolar reflectivity quite well, but fail to reproduce the expected polarimetric signature.
Status summer 2024
During the first year, we aimed to implement the DDA-based scattering module into the EMVORADO forward operator. The focus is on the consistency between the ICON model's microphysical assumptions, where we first concentrate on the 2-moment microphysics scheme (Seifert and Beheng, 2006) and the forward simulations. This allows direct comparison with the legacy T-matrix simulations, hence an immediate assessment of the impact of the scattering model. Also, the DDA single-scattering properties of individual ice and snow particles are available.
Contribution of University of Cologne
The first goal of the University of Cologne's contribution to PRISTINE was to develop a scattering database for realistically shaped ice and snow particles for different radar frequencies (C-, X-, Ka-, and W-band as those are the ones in use in polarimetric weather and cloud radars in Germany) and to provide DWD with a ready-to-use database of azimuthally-averaged polarimetric scattering properties over a set of radar elevation angles and canting angles for each particle.
Unlike the T-matrix, DDA (Discrete Dipole Approximation, Yurkin and Hoekstra, 2007) is an advanced scattering model that can simulate scattering properties from irregularly shaped particles. This is obtained by representing the complex shapes of particles with a set of cubic voxels distributed in space. By increasing the resolution of such representation (i.e. reducing the size length of the cubes), it is possible to describe the complex geometrical details of small ice crystals.
Firstly, we simulated realistically shaped cloud ice particles (i.e., single crystals, monomers) including plates, simply defined by their geometrical properties and representing crystals up to sizes for 0.5 mm, and dendrite shapes using Reiter's algorithm (Reiter, 2005), representing larger crystals. Figure 1 shows a few examples of simulated realistic dendrites and plates.
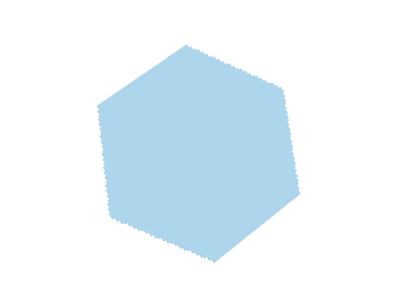
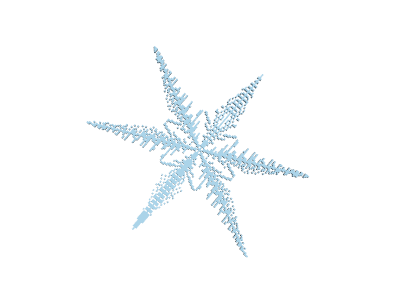
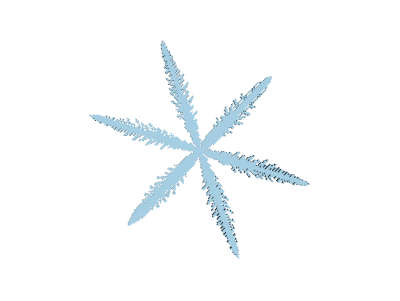
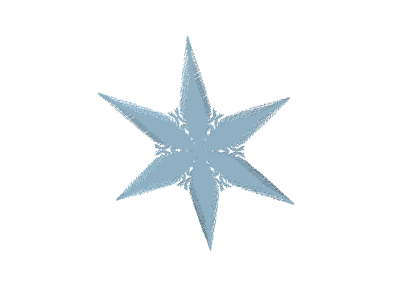

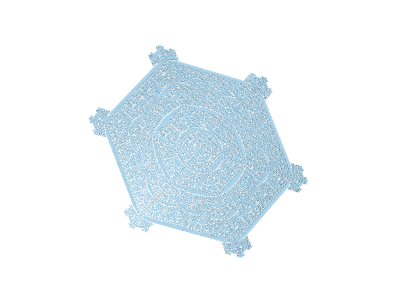
As the focus is on the consistency between the microphysical assumptions made in the ICON model and the forward simulations, we determine the aspect ratio of the simulated monomers to match the ICON mass-size relationship. Figure 2 shows the resulting aspect ratios over particle size for different shapes of dendrites. With increasing size, aspect ratio, in general, decreases. The large variability of aspect ratio at lower sizes is caused by using a constant resolution (20um) for all the particles. However, we are now revisiting our strategy and adapting the resolution to homogenize the representativity of particle shapes at various size ranges.
Further, we use an aggregation model to generate aggregates using the simulated plates and dendrites. Figure 3 shows the mass-size relation for the generated aggregates and the ICON 2mom snow microphysics. Since snow aggregation is a stochastic process, it is not straightforward to control the size and mass of the resulting aggregate as it was for the individual crystals. For this reason, we generated a lot of aggregate shapes varying the parameters of the aggregation process (namely, the number and size distribution of the colliding crystals that end up composing the aggregate). For the smallest aggregate size, the icon assumptions require a far too dense aggregate shape that wasn’t possible to model using realistic assumptions. For this portion of the size spectrum we plan to use again single crystals. For the larger sizes, we get some aggregates that fall on the ICON 2moment snow mass-size relation. We select those aggregates that best fit the ICON 2-moment mass-size relation to proceed further with the scattering calculation. The ICON size limit for the snow class is 0.05 mm to 50 mm. We divided the size range into 256 linear-size bins and simulated snow aggregates up to 13 mm so far. The selection criteria first consisted of in a mass-threshold that discarded all shapes with masses that were more than 10% away from the assumed relation. Then we selected for each size bin the shape that had it maximum dimension closest to the bin center.
DDA considers each cube representing the scattering particle as a discrete polarizable element (originally assumed to be an electric dipole, hence the name DDA). The model simulates the interaction of all these polarizable elements with an incident electromagnetic wave and among all the elements. The superposition of the resulting radiated electric field allows to calculate the scattering properties of the particle. We used DDA to compute the forward and backward scattering properties for each of the selected ice and snow particles for multi-frequency radar bands. Further, we calculate the azimuthal orientation average for each particle from the DDA simulated scattering properties and provide the data to DWD for implementation in EMVORADO. The azimuthal orientation average for the C-band was calculated for the first result. while the scattering calculations for the X, Ka and W bands are currently running.
Figure 3: Green line represents the mass-size relation of snow in the ICON 2-moment microphysics. Blue dots represent masses of the simulated aggregates, and the yellow dots highlight those aggregate masses within 10% of the ICON snow mass.
We plan to use McSnow, a Lagrangian particle-based model (Brdar and Seifert, 2018) that can help us get more realistic ice particles. We can get more realistic snow shapes in conjunction with the aggregation model that we used before. Getting particle structures by using a combination of a Lagrangian super-particle model and an aggregation model rather than from empirical and idealized habits will allow an evaluation of the uncertainties of the computed scattering properties that arise from the variety of snow particle shapes, which are unknown in operational microphysical schemes. As our focus is to keep consistency with microphysical assumptions made in the ICON model, we are currently evaluating the discrepancies of ice and snow microphysical properties between McSnow and ICON when simulating the same cloud scene. Also, the DDA scattering calculations with varying resolution particles are ongoing.
Contribution of Deutscher Wetterdienst
The operational version of the EMVORADO forward operator so far offers to calculate hydrometeor scattering properties from the Rayleigh, Mie, and T-matrix methods. For efficiency, Mie and T-matrix options allow to apply lookup tables of bulk scattering properties that are internally pre-calculated per hydrometeor class. In PRISTINE, we extend this procedure with lookup tables based on externally calculated scattering properties.
Based on the ready-to-use data of azimuthally-averaged polarimetric scattering properties of individual hydrometeors provided by the University of Cologne, we produce EMVORADO-equivalent polarimetric bulk scattering lookup tables. In this first stage, we apply external, python-based tools to perform the integrations over the hydrometeor-class specific particle size (or mass) distributions, consistently with ICON microphysics, and particle orientation distributions. These lookup tables are then used by EMVORADO in the same way as the ones internally derived from Mie or T-matrix calculations. In a later step, the size and orientation integration procedures will be performed within EMVORADO consistently with those of the other scattering methods, and the lookup tables will be produced internally from externally calculated single particle scattering properties like those produced by the University of Cologne.
Figure 4: Equivalent ZH, ZDR, and KDP from EMVORADO cloud ice bulk scattering lookup tables from operational internal T-matrix calculations and external DDA-based scattering data from the PRISTINE project. Note that at this state, data for small particles (<0.5mm) is lacking, hence results for small Dmean should be taken with care.
So far, lookup tables have been produced for cloud ice, i.e. from single crystals scattering properties, illustrated in Figure 4 in the form of radar equivalents in the C-band in comparison to the corresponding (i.e., same underlying size and orientation distributions) legacy T-matrix data. Reflectivity (ZH) equivalents from T-matrix and DDA scattering calculations are very similar, as expected. Differential reflectivity (ZDR) and specific differential phase (KDP), however, exhibit very different characteristics regarding their dependency on mean particle size in the bulk. While the T-matrix data shows high ZDR and KDP for small mean sizes, maximum values for DDA data occur at large mean sizes. These discrepancies are critical in many applications, like model evaluation or data assimilation, as they will lead to different results and conclusions, e.g., regarding the question of whether the model predicts too large or too small particles. This underlines again the importance of applying more realistic hydrometeor shapes in scattering calculations.
Figure 5: Observed (left) as well as T-matrix (middle) and DDA (right) based simulated QVP of ZDR (top) and KDP (bottom) from the 12° elevation scan over DWD C-band radar station Prötzel on 25 July 2017. Blue and yellow dashed boxes indicate the cloud ice and snow dominated layers, respectively.
The DDA-based cloud ice lookup tables have then been applied in EMVORADO simulations. One case day from the Operation Hydrometeors project with primarily stratiform situations has been used and simulations of the radar observations of the DWD C-band radar network performed based on assimilation-aided ICON forecasts. Time-height displays of quasi-vertical profiles (QVPs) of observed and of simulated T-matrix and DDA-based ZDR and KDP are shown in Figure 5.
In the upper, cloud ice dominated layers (above ~6 km), simulations from T-matrix data slightly overestimated ZDR, and simulations from DDA data show significant overestimations. KDP was underestimated with the T-matrix data; the agreement improved with the DDA scattering data, but an underestimation still persists. Replacing the low-density proxies (soft spheroids in T-matrix calculations) with realistically shaped particles, an increase, particularly in ZDR, was to be expected (e.g., Schrom and Kumjian, 2018). The disagreement with the observations might be both due to the model ICON (e.g., predicting too large crystals as, e.g., also suggested by the microphysical retrievals performed in PROM-Operation Hydrometeors and PROM-PARA, see e.g., (Trömel et al., 2023) and the forward operator EMVORADO (e.g., the too strong orientation of the crystals assumed), and further analysis is ongoing.
Because only scattering data for realistically shaped cloud ice, but not for snow, was applied so far, little change is observed in the simulations’ “polarimetric void” layer between 3 – 6 km, where snow dominates. This issue will be tackled in the next step when DDA scattering data for aggregate particles becomes available.
References
- Brdar, S. and A. Seifert, 2018: McSnow: A Monte-Carlo Particle Model for Riming and Aggregation of Ice Particles in a Multidimensional Microphysical Phase Space, J. Adv. Model. Earth Syst.,10, 187–206.
- Reiter, C. A., 2005: A local cellular model for snow crystal growth Chaos, Solitons & Fractals, 23 (4), pp. 1111-1119.
- Schrom, R.S. and M. R. Kumjian, 2018: Bulk-density representations of branched planar ice crystals: errors in the polarimetric radar variables, J Appl Meteor Climatol., 57(2), 333–346.
- Seifert, A. and K. Beheng, 2006: A two-moment cloud microphysics parameterization for mixed-phase clouds. Part 1: Model description. Meteorol. Atmos. Phys. 92, 45–66.
- Trömel, S., U. Blahak, R. Evaristo, J. Mendrok, L. Neef, V. Pejcic, T. Scharbach, P. Shrestha, and C. Simmer, 2023: Fusion of radar polarimetry and atmospheric modeling, In: Bringi, V. N. , Mishra, K.V., and Thurai, M. (Eds.): Advances in Weather Radar, Volume 2: Precipitation science, scattering and processing algorithms, 293–344.
- Yurkin, M. A. and A. G. Hoekstra, 2007: The discrete dipole approximation: An overview and recent developments, J Quant Spectrosc Radiat Transf, 106, pp. 558-589.